by Paul Gilster | Dec 14, 2021 | Exoplanetary Science |
The day is not far off when we’ll be able to look at a small planet in the habitable zone of its star and detect basic features on its surface: water, ice, land. The era of the 30-meter extremely large telescope approaches, so this may even be possible from the ground, and large space telescopes will be up to the challenge as well (which is why things like aperture size and starshade prospects loom large in our discussions of current policy decisions).
Consider this: On the Earth, while the atmosphere reflects a huge amount of light from the Sun, about half the total albedo at the poles comes from polar ice. It would be useful, then, to know more about the ice and land distribution that we might find on planets around other stars. This is the purpose of a new paper in the Planetary Science Journal recounting the creation of climate simulations designed to predict how surface ice will be distributed on Earth-like exoplanets. It’s a relatively simple model, the authors acknowledge, but one that allows rapid calculation of climate on a wide population of hypothetical planets.
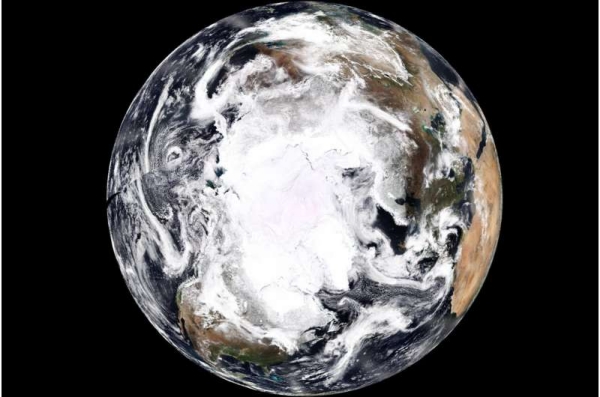
Image: A composite of the ice cap covering Earth’s Arctic region — including the North Pole — taken 512 miles above our planet on April 12, 2018 by the NOAA-20 polar-orbiting satellite. Credit: NOAA.
Lead author Caitlyn Wilhelm (University of Washington) began the work while an undergraduate; she is now a research scientist at the university’s Virtual Planet Laboratory:
“Looking at ice coverage on an Earth-like planet can tell you a lot about whether it’s habitable. We wanted to understand all the parameters—the shape of the orbit, the axial tilt, the type of star—that affect whether you have ice on the surface, and if so, where.”
Thus we attempt to cancel out imprecision in the energy balance model (EBM) the paper deploys by sheer numbers, looking for general patterns like the fraction of planets with ice coverage and the location of their icy regions. A ‘baseline of expectations’ emerges for planets modeled to be like the Earth (which in this case means a modern Earth), worlds of similar mass, rotation, and atmospherics. The authors simulate more than 200,000 such worlds in habitable zone orbits.
What is being modeled here is the flow of energy between equator and pole as it sets off climate possibilities for the chosen population of simulated worlds over a one million year timespan. These are planets modeled to be in orbit around stars in the F-, G- and K-classes, which takes in our G-class Sun, and all of them are placed in the habitable zone of the host star. The simulations take in circular as well as eccentric orbits, and adjust axial tilt from 0 all the way to 90 degrees. By way of contrast, Earth’s axial tilt is 23.5 degrees. That of Uranus is close to 90 degrees. The choice of axial tilt obviously drives extreme variations in climate.
But let’s pause for a moment on that figure I just gave: 23.5 degrees. Because factors like this are not fixed, and Earth’s obliquity, the tilt of its spin axis, actually isn’t static. It ranges between roughly 22 degrees and 24.5 degrees over a timescale of some 20,000 years. Nor is the eccentricity of Earth’s orbit fixed at its current value. Over a longer time period, it ranges between a perfectly circular orbit (eccentricity = zero) to an eccentricity of 6 percent. While these changes seem small enough, they have serious consequences, such as the ice ages.
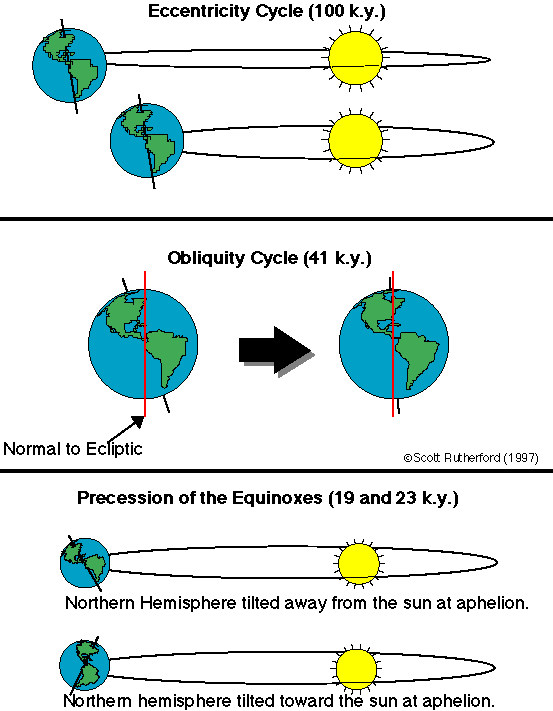
Image: The three main variations in Earth’s orbit linked to Milankovitch cycles. The eccentricity is the shape of Earth’s orbit; it oscillates over 100,000 years (or 100 k.y.). The obliquity is the tilt of Earth’s spin axis, and the precession is the alignment of the spin axis. Credit: Scott Rutherford. A good entry into all this is Sean Raymond’s blog planetplanet, where he offers an exploration of life-bearing worlds and the factors that influence habitability. An astrophysicist based in Bordeaux, Raymond’s name will be a familiar one to Centauri Dreams readers. I should add that he is not involved in the paper under discussion today.
Earth’s variations in orbit and axial tilt are referred to as Milankovitch cycles, after Serbian astronomer Milutin Milankovi?, who examined these factors in light of changing climatic conditions over long timescales back in the 1920s. These cycles can clearly bring about major variations in surface ice as their effects play out. If this is true of Earth, we would expect a wide range of climates on planets modeled this way, everything from hot, moist conditions to planet-spanning ‘snowball’ scenarios of the sort Earth once experienced.
So it’s striking that even with all the variation in orbit and axial tilt and the wide range in outcomes, only about 10 percent of the planets in this study produced even partial ice coverage. Rory Barnes (University of Washington) is a co-author of the paper:
“We essentially simulated Earth’s climate on worlds around different types of stars, and we find that in 90% of cases with liquid water on the surface, there are no ice sheets, like polar caps. When ice is present, we see that ice belts—permanent ice along the equator—are actually more likely than ice caps.”
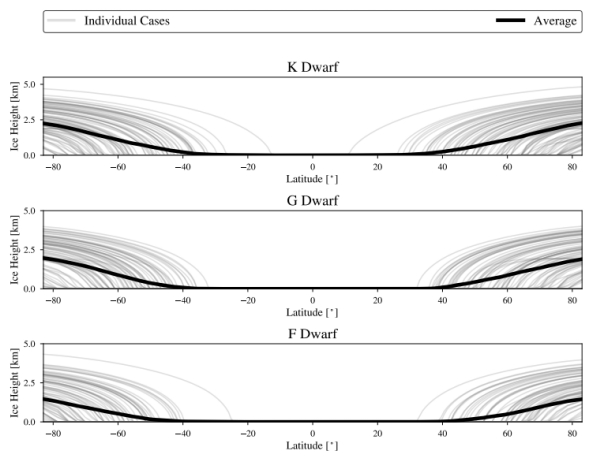
Image. This is Figure 12 from the paper. Caption: Figure 12. Range and average ice heights of ice caps as a function of latitude for stars orbiting F (top), G (middle) and K (bottom) dwarf stars. Note the different scales of the x-axes. Light grey curves show 100 randomly selected individual simulations, while black shows the average of all simulations that concluded with an ice belt. Although the averages are all symmetric about the poles, some individual ice caps are significantly displaced. Credit: Wilhelm et al.
Breaking this down, the authors show that in their simulations, planets like Earth are most likely to be ice-free. Even oscillations in orbital eccentricity and axial tilt do not prevent, however, planets orbiting the F-, G- and K-class stars in the study from developing stable ice belts on land. Moreover, ice belts turn out to be twice as common as polar ice caps for planets around G- and K-class stars. As to size, the typical extension of an ice belt is between 10 and 30 degrees, varying with host star spectral type, and this is a signal large enough to show up in photometry and spectroscopy, making it a useful observable for future instruments.
This is a study that makes a number of assumptions in the name of taking a first cut at the ice coverage question, each of them “…made in the name of tractability as current computational software and hardware limitations prevent the broad parameter sweeps presented here to include these physics and still be completed in a reasonable amount of wallclock time. Future research that addresses these deficiencies could modify the results presented above.”
Fair enough. Among the factors that will need to be examined in continued research, all of them spelled out here, are geochemical processes like the carbonate-silicate cycle, ocean heat transport as it affects the stability of ice belts, zonal winds and cloud variability, all of this not embedded in the authors’ energy balance model, which is idealized and cannot encompass the entire range of effects. Nor do the authors simulate the frequency and location of M-dwarf planet ice sheets.
But the finding about the lack of ice in so many of the simulated planets remains a striking result. Let me quote the paper’s summation of the findings. They remove the planets ending in a moist greenhouse or snowball planet, these worlds being “by definition, uninhabitable.” We’re left with this:
…we then have 39,858 habitable F dwarf planets, 37,604 habitable G dwarf planets, and 36,921 habitable K dwarf planets in our sample. For G dwarf planets, the ice state frequencies are 92% ice free, 2.7% polar cap(s), and 4.8% ice belt. For F dwarf planets, the percentages are 96.1%, 2.9%, and 0.9%, respectively. For K dwarf planets, the percentages are 88.4%, 3.5%, and 7.6%, respectively. Thus, we predict the vast majority of habitable Earth-like planets of FGK stars will be ice free, that ice belts will be twice as common as caps for G and K dwarfs planets, and that ice caps will be three times as common as belts for Earth-like planets of F dwarfs.
And note that bit about the uninhabitability of snowball worlds, which the paper actually circles back to:
Our dynamic cases highlight the importance of considering currently ice-covered planets as potentially habitable because they may have recently possessed open surface water. Such worlds could still develop life in a manner similar to Earth, e.g. in wet/dry cycles on land, but then the dynamics of the planetary system force the planet into a snowball, which in turn forces life into the ocean under a solid ice surface. Such a process may have transpired multiple times on Earth, so we should expect similar processes to function on exoplanets.
The paper is Wilhelm et al., ”The Ice Coverage of Earth-like Planets Orbiting FGK Stars,” accepted at the Planetary Science Journal (preprint). Source code available. Scripts to generate data and figures also available.
by Paul Gilster | Dec 10, 2021 | Astrobiology and SETI |
If we ever do receive a targeted message from another star – as opposed to picking up, say, leakage radiation – will we be able to decipher it? We can’t know in advance, but it’s a reasonable assumption that any civilization wanting to communicate will have strategies in place to ease the process. In today’s essay, Brian McConnell begins a discussion on SETI and interstellar messaging that will continue in coming weeks. The limits of our understanding are emphasized by the problem of qualia; in other words, how do different species express inner experience? But we begin with studies of other Earth species before moving on to data types and possible observables. A communication systems engineer and expert in translation technology, Brian is the author of The Alien Communication Handbook — So We Received A Signal, Now What?, recently published by Springer Nature under their Astronomer’s Bookshelf imprint, and available through Amazon, Springer and other booksellers.
by Brian McConnell
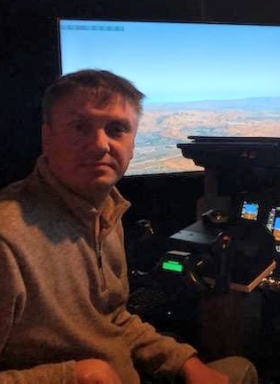
Animal Communication
What do our attempts to understand animal communication have to say about our future efforts to understand an alien transmission or information-bearing artifact, should we discover one? We have long sought to communicate with “aliens” here on Earth. The process of deciphering animal communication has many similarities with the process of analyzing and comprehending an ET transmission, as well as important differences. Let’s look at the example of audio communication among animals, as this is analogous to a modulated electromagnetic transmission.
The general methodology used is to record as many samples of communication and behavior as possible. This is one of the chief difficulties in animal communication research, as the process of collecting recordings is quite labor intensive, and in the case of animals that roam over large territories it may be impossible to observe them in much of their environment. Animals that have a small territory where they can be observed continuously are ideal.
Once these observations are collected, the next step is to understand the basic elements of communication, similar to phonemes in human speech or the letters in an alphabet. This is a challenging process as many animals communicate using sounds outside the range of human hearing, and employ sounds that are very different from human speech. This typically involves studying time versus frequency plots of audio recordings, to understand the structure of different utterances, which is also very labor intensive. This is one area where AI or deep learning can help greatly, as AI systems can be designed to automate this step, though they require a large sample corpus to be effective.
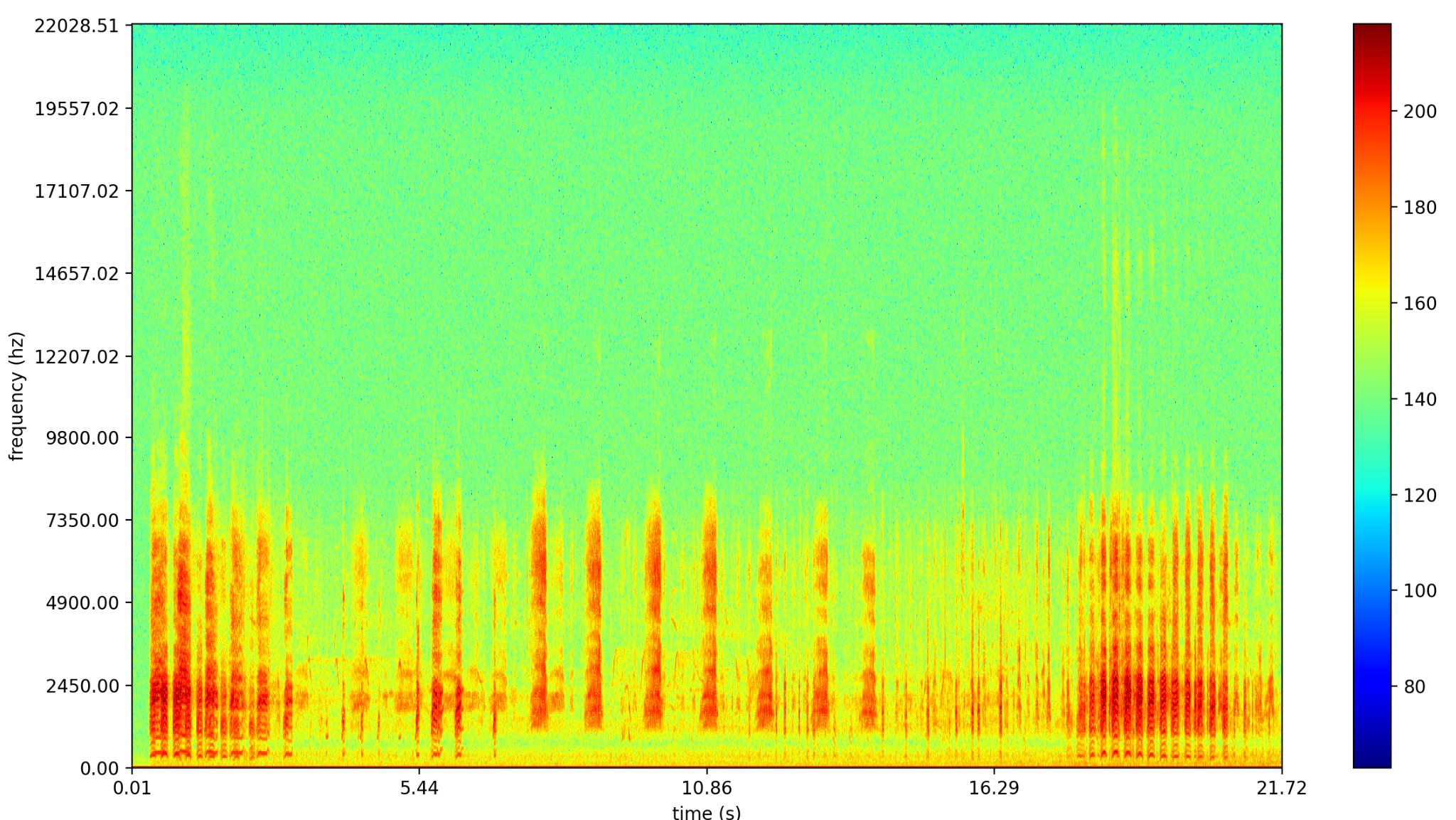
Time vs frequency plot of duck calls (click to enlarge).Credit: Brian McConnell.
The next step, once the basic units of communication are known, is to use statistical methods to understand how frequently they are used in conjunction with each other, and how they are grouped together. Zipf’s Law is an example of one method that can be used to understand the sophistication of a communication system. In human communication, we observe that the probability of a word being used is inversely proportional to its overall rank.
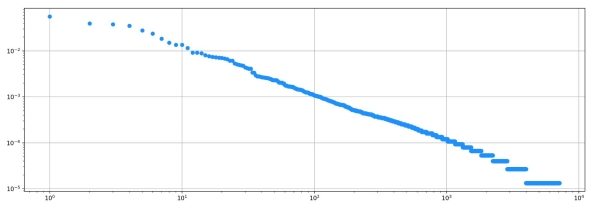
A log-log plot of the frequency of word use (y axis) versus word rank (x axis) from the text of Mary Shelley’s Frankenstein. Notice that the relationship is almost exactly 1/x. Image credit: Brian McConnell, The Alien Communication Handbook.
Conditional probability is another target for study. This refers to the probability that a particular symbol or utterance will follow another. In English, for example, letters are not used with equal frequency, and some pairs or triplets of letters are encountered much more often than others. Even without knowing what an utterance or group of utterances means, it is possible to understand which are used most often, and are likely most important. It is also possible to quantify the sophistication of the communication system using methods like this.
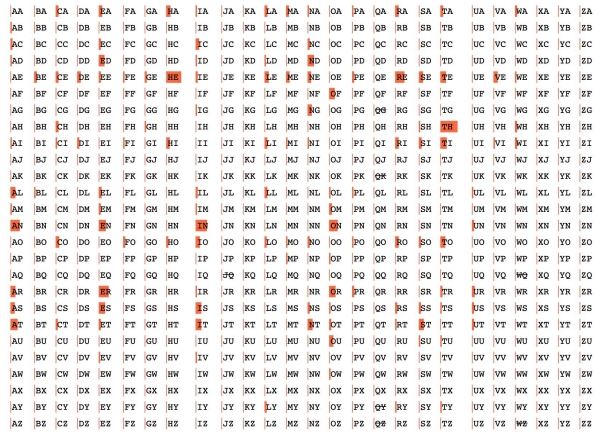
A graph of the relative frequency of use of bigrams (2 letter combinations) in English text (click to enlarge). You can see right away that some bigrams are used extensively while others very rarely occur.. Credit: Peter Norvig.
With this information in hand, it is now possible to start mapping utterances or groups of utterances to meanings. The best example of this to date is Con Slobodchikoff ’s work with prairie dogs. They turned out to be an ideal subject of study as they live in colonies, known as towns, and as such could be observed for extended periods of time in controlled experiments. Con and his team observed how their calls differed as various predators approached the town, and used a solve for x pattern to work out which utterances had unique meanings.
Using this approach, in combination with audio analysis, Con and his team worked out that prairie dogs had unique “words” for humans, coyotes and dogs, as well as modifiers (adjectives) such as short, tall, fat, thin, square shaped, oval shaped and carrying a gun. They did this by monitoring how their chirps varied as different predators approached, or as team members walked through with different color shirts, etc. They also found that the vocabulary of calls varied in different towns, which suggested that the communication was not purely instinctual but had learned components (cultural transmission). While nobody would argue that prairie dogs communicate at a human level, their communication does appear to pass many of the tests for language.
The challenge in understanding communication is that unless you can observe the communication and a direct response to something, it is very difficult to work out its meaning. One would presume that if prairie dogs communicate about predators, they communicate about other less obvious aspects of their environment that are more challenging to observe in controlled experiments. The problem is that this is akin to listening to a telephone conversation and trying to work out what is being said only by watching how one party responds.
Research with other species has been even more limited, mostly because of the twin difficulties of capturing a large corpus of recordings, along with direct observations of behavior. Marine mammals are a case in point. While statistical analysis of whale and dolphin communication suggests a high degree of sophistication, we have not yet succeeded in mapping their calls to specific meanings. This should improve with greater automation and AI based analysis. Indeed, Project CETI (Cetacean Translation Initiative) aims to use this approach to record a large corpus of whale codas and then apply machine learning techniques to better understand them.
That our success in understanding animal communication has been so limited may portend that we will have great difficulty in understanding an ET transmission, at least the parts that are akin to natural communication.
The success of our own communication relies upon the fact that we all have similar bodies and experiences around which we can build a shared vocabulary. We can’t assume that an intelligent alien species will have similar modes of perception or thought, and if they are AI based, they will be truly alien.
On the other hand, a species that is capable of designing interstellar communication links will also need to understand information theory and communication systems. An interstellar communication link is essentially an extreme case of a wireless network. If the transmission is intended for us, and they are attempting to communicate or share information, they will be able to design the transmission to facilitate comprehension. That intent is key. This is where the analogy to animal communication breaks down.
Observables
An important aspect of a well designed digital communication system is that it can interleave many different types of data or media types. Photographs are an example of one media type we may be likely to encounter. A civilization that is capable of interstellar communication will, by definition, be astronomically literate. Astronomy itself is heavily dependent on photography. This isn’t to say that vision will be their primary sense or mode of communication, just that in order to be successful at astronomy, they will need to understand photography. One can imagine a species whose primary sense is via echolocation, but has learned to translate images into a format they can understand, much as we have developed ultrasound technology to translate sound into images.
Digitized images are almost trivially easy to decode, as an image can be represented as an array of numbers. One need only guess the number of bits used per pixel, the least to most significant bit order, and one dimension of the array to successfully decode an image. If there are multiple color channels, there are a few additional parameters, but even then the parameter space is very small, and it will be possible to extract images if they are there. There are some additional encoding patterns to look for, such as bitplanes, which I discuss in more detail in the book, but even then the number of combinations to cycle through remains small.
The sender can help us out even further by including images of astronomical objects, such as planets, stars and distant nebulae. The latter are especially interesting because they can be observed by both parties, and can be used to guide the receiver in fine calibrations, such as the color channels used, scaling factors (e.g. gamma correction), etc. Meanwhile, images of planets are easy to spot, even in a raw bitstream, as they usually consist of a roundish object against a mostly black background.
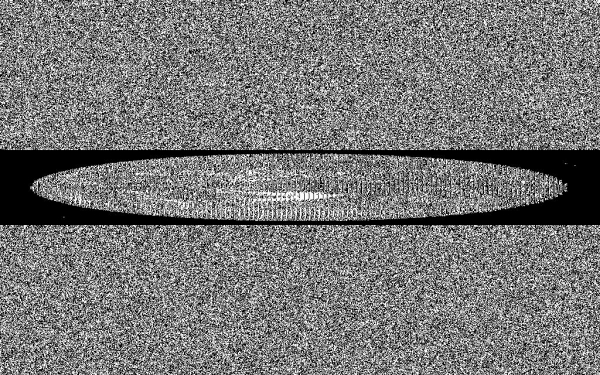
An example of a raw bitstream that includes an image of a planet amid what appears to be random or efficiently encoded data. All the viewer needs to do to extract the image is to work out one dimension of the array along with the number of bits per pixel. The degree to which a circular object is stretched into an ellipse also hints at the number of bits per pixel. Credit: Brian McConnell, The Alien Communication Handbook.
What is particularly interesting about images is that once you have worked out the basic encoding schemes in use, you can decode any image that uses that encoding scheme. Images can represent scenes ranging from microscopic to cosmic scales. The sender could include images of anything, from important landmarks or sites to abstract representations of scenes (a.k.a. art). Astute readers will notice that these are uncompressed images, and that the sender may wish to employ various compression schemes to maximize the information carrying capacity of the communication channel. Compressed images will be much harder to recognize, but even if a relatively small fraction of images are uncompressed, they will stand out against what appears to be random digits, as in the example bitstream above.
Semantic Networks
The sender can take this a step further by linking observables (images, audio samples) with numeric symbols to create a semantic network. You can think of a semantic network like an Internet of ideas, where each unique idea has a numeric address. What’s more, the address space (the maximum number of ideas that can be represented) can be extremely large. For example, a 64 bit address space has almost 2 x 1019 unique addresses.
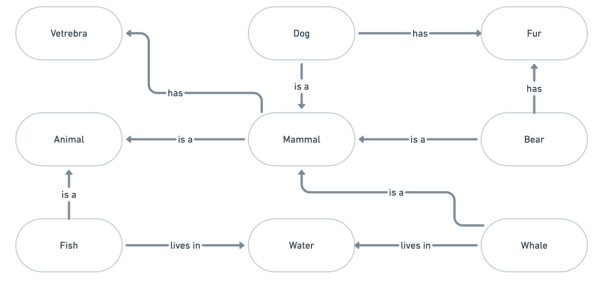
An example of a semantic network representing the relationship between different animals and their environment (click to enlarge). The network is shown in English for readability but the nodes and the operators that connect them could just as easily be based on a numeric address space.
The network doesn’t need to be especially sophisticated to enable the receiver to understand the relationships between symbols. In fact, the sender can employ a simple way of saying “This image contains the following things / symbols” by labeling them with one or more binary codes within the images themselves.
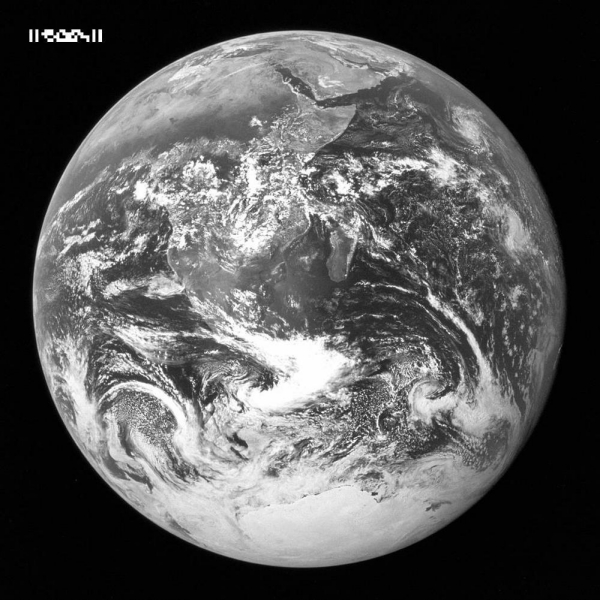
An example of an image that is labeled with four numeric codes representing properties within the image. Credit: Brian McConnell, The Alien Communication Handbook.
Observables Versus Qualia
While this pattern can be used to build up a large vocabulary of symbols that can be linked to observables (images, audio samples, and image sequences), it will be difficult to describe qualia (internal experiences). How would you describe the concept of sweetness to someone who can’t experience a sweet taste? You could try linking the concept to a diagram of a sugar molecule, but would the receiver make the connection between sugar and sweetness? Emotional states such as fear and hunger may be similarly difficult to convey. How would you describe the concept of ennui?
Imagine an alien species whose nervous system is more decentralized like an octopus. They might have a whole vocabulary around the concept of “brain lock”, where different sub brains can’t reach agreement on something. Where would we even start with understanding concepts like this? It’s likely that while we might be successful in understanding descriptions of physical objects and processes, and that’s not nothing, we may be flummoxed in understanding descriptions of internal experiences and thoughts. This is something we take for granted in human language, primarily because even with differences in language, we all share similar bodies and experiences around which we build our languages.
Yet all hope is not lost. Semantic networks allow a receiver to understand how unknown symbols are related to each other, even if they don’t understand their meaning directly. Let’s consider an example where the sender is defining a set of symbol codes we have no direct understanding of, but we have previously figured out the meaning of symbol codes that define set membership (?), greater/lesser in degree (<>), and oppositeness (?) .
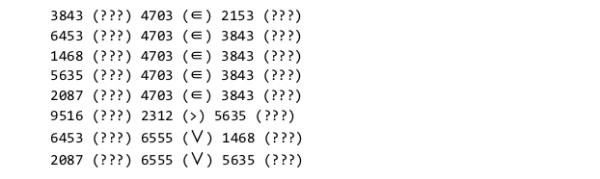
Even without knowing the meaning of these new symbol codes, the receiver can see how they are related and can build a graph of this network. This graph in turn can guide the receiver in learning unknown symbols. If a symbol is linked to many others in the network, there may be multiple paths toward working out its meaning in relation to symbols that have been learned previously. Even if these symbols remain unknown, the receiver has a way of knowing what they don’t know, and can map their progress in understanding.
The implication for a SETI detection is that we may find it is both easier and more difficult to understand what they are communicating than one may expect. Objects or processes that can be depicted numerically via images, audio or image sequences may enable the formation of a rich vocabulary around them and with relative ease, while communication around internal experiences, culture, etc may remain partially understood at best.
Even partial comprehension based on observables will be a significant achievement, as it will enable the communication of a wide range of subjects. And as can be shown, this can be done with static representations. An even more interesting scenario is if the transmission includes algorithms, functions from computer programs. Then it will be possible for the receiver to interact with them in real time, which enables a whole other realm of possibilities for communication.
More on that in the next article…
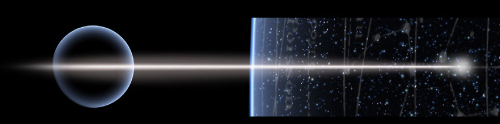
by Paul Gilster | Dec 9, 2021 | Outer Solar System |
A spray of organic molecules and ice particles bursting out of an outer system moon is an unforgettable sight, as Cassini showed us at Enceladus. Finding something similar at Europa would be a major help for future missions there, given the opportunity to sample a subsurface ocean that is perhaps as deep as 160 kilometers. But Lynnae Quick (NASA GSFC), who works on the science team that produced the Europa Imaging System cameras that will fly on the Europa Clipper mission, offers a cautionary note:
“A lot of people think Europa is going to be Enceladus 2.0, with plumes constantly spraying from the surface. But we can’t look at it that way; Europa is a totally different beast.”
A good thing that Europa Clipper can produce evidence of conditions beneath the ice without the need for plumes when it begins its explorations in 2031. In fact, adds Quick, every instrument aboard the spacecraft has its own role to play in the study of that global ocean. Still, potential plumes are too important to ignore, even if finding an active, erupting Europa would by no means be as straightforward as discovering the plumes of Enceladus. The Europa evidence we have indicates faint plume activity through Galileo and Hubble data and some Earth-based telescopes.
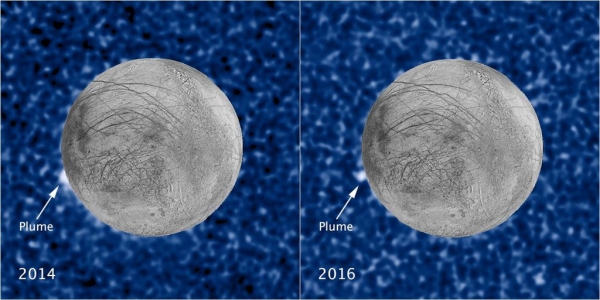
Image: These composite images show a suspected plume of material erupting two years apart from the same location on Jupiter’s icy moon Europa. The images bolster evidence that the plumes are a real phenomenon, flaring up intermittently in the same region on the satellite. Both plumes, photographed in ultraviolet light by NASA’s Hubble’s Space Telescope Imaging Spectrograph, were seen in silhouette as the moon passed in front of Jupiter. Credit: NASA/JPL.
In the image above, notice the possible plume activity. At left is a 2014 event that appears in Hubble data, a plume estimated to be 50 kilometers high. At the right, and in the same location, is an image taken two years later by the same Hubble Imaging Spectrograph, both events seen in silhouette as the moon passed in front of Jupiter. It’s noteworthy that this activity occurs at the same location as an unusually warm spot in the ice crust that turned up in Galileo mission data from the 1990s.
Let’s now cut to a second image, showing that Galileo find. Below we see the surface of Europa, focusing on what NASA calls a ‘region of interest.’
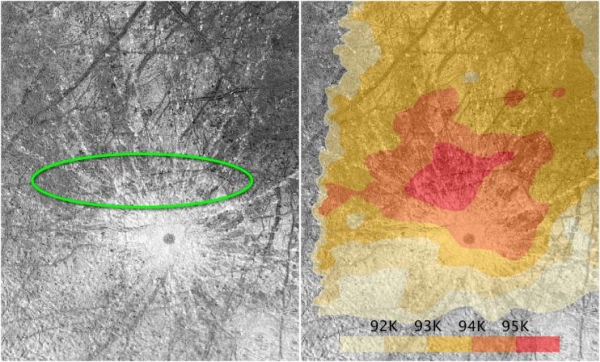
Image: The image at left traces the location of the erupting plumes of material, observed by NASA’s Hubble Space Telescope in 2014 and again in 2016. The plumes are located inside the area surrounded by the green oval. The green oval also corresponds to a warm region on Europa’s surface, as identified by the temperature map at right. The map is based on observations by the Galileo spacecraft. The warmest area is colored bright red. Researchers speculate these data offer circumstantial evidence for unusual activity that may be related to a subsurface ocean on Europa. The dark circle just below center in both images is a crater and is not thought to be related to the warm spot or the plume activity. Credit:
NASA/ESA/W. Sparks (STScI)/USGS Astrogeology Science Center.
Getting access to the realm below the surface would obviate the need to drill through kilometers of ice in some future mission, giving us a better understanding of possible habitability. An ocean churned by activity from heated rock below the seafloor could spawn the kind of life we find around hydrothermal vents here on Earth, circulating carbon, hydrogen, oxygen, nitrogen, phosphorus, and sulfur deep within. Moreover, Europa is in an elliptical orbit that generates internal heat and likely drives geology.
Does an icy plate tectonics also exist on this moon? The Europan surface is laced with cracks and ridgelines, with surface blocks having apparently shifted. Bands that show up in Galileo imagery delineate zones where fresh material from the underlying shell appears to have moved up to fill gaps as soon as they appear. A 2014 paper (citation below) by Simon Kattenhorn (University of Idaho – Moscow) and Louise Prockter (JHU/APL) found evidence of subduction in Galileo imagery, where one icy plate seems to have moved beneath another, forcing surface material into the interior.
That paper is, in fact, worth a quote. The italics are mine:
…we produce a tectonic reconstruction of geologic features across a 134,000 km2 region of Europa and find, in addition to dilational band spreading, evidence for transform motions along prominent strike-slip faults, as well as the removal of approximately 20,000 km2 of the surface along a discrete tabular zone. We interpret this zone as a subduction-like convergent boundary that abruptly truncates older geological features and is flanked by potential cryolavas on the overriding ice. We propose that Europa’s ice shell has a brittle, mobile, plate-like system above convecting warmer ice. Hence, Europa may be the only Solar System body other than Earth to exhibit a system of plate tectonics.
This is an encouraging scenario in which surface nutrients produced through interactions with radiation from Jupiter are driven into pockets in the ice shell and perhaps into the ocean below, even as chemical activity continues at the seafloor. If we find plumes, their chemical makeup could put these scenarios to the test. But as opposed to the highly visible plumes of Enceladus, any Europan plumes would be harder to detect, bound more tightly to the surface because of the higher Europan gravity, and certainly lacking the spectacular visual effects at Enceladus.
Key to the search for plumes will be Clipper’s EIS camera suite, which can scout for activity at the surface by scanning the limb of the moon as it passes in front of Jupiter. Moreover, a plume should leave a deposit on surface ice that EIS may see. Clipper’s Europa Ultraviolet Spectrograph (Europa-UVS) will look for plumes at the UV end of the spectrum, tracking the chemical makeup of any that are detected. The Europa Thermal Emission Imaging System (E-THEMIS) will be able to track hotspots that may indicate recent eruptions. A complete description of Clipper’s instrument suite is available here.
We’ve been using Galileo data for a long time now. It’s a refreshing thought that we’ll have two spacecraft – Europa Clipper and Jupiter Icy Moons Explorer (JUICE) – in place in ten years to produce what will surely be a flood of new discoveries.
The paper on Europan plate tectonics is Kattenhorn & Prockter, “Evidence for subduction in the ice shell of Europa,” Nature Geoscience 7 (2014), 762-767 (abstract).
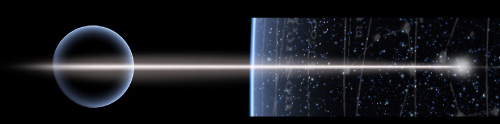
by Paul Gilster | Dec 7, 2021 | Astrobiology and SETI |
I always knew where I stood with Alexander Zaitsev. In the period 2008-2011, he was a frequent visitor on Centauri Dreams, drawn initially by an article I wrote about SETI, and in particular whether it would be wise to go beyond listening for ETI and send out directed broadcasts to interesting nearby stars. At that time, I was straddling the middle on METI — Messaging to Extraterrestrial Intelligence — but Dr. Zaitsev found plenty of discussion here on both sides, and he joined in forcefully.
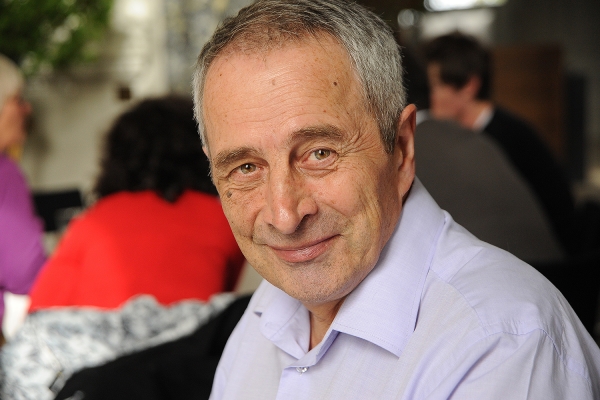
Image: Alexander Leonidovich Zaitsev, METI advocate and radio astronomer, whose messages to the cosmos include the 1999 and 2003 ‘Cosmic Calls’ from Evpatoria. Credit: Seth Shostak.
The Russian astronomer, who died last week, knew where he stood, and he knew where you should stand as well. As my own views on intentional broadcasts moved toward caution in future posts, he and I would have the occasional email exchange. He was always courteous but sometimes exasperated. When I was in his good graces, his messages would always be signed ‘Sasha.’ When he was feeling combative, they would be signed ‘Alexander.’ And if I really tripped his wires, they would end with a curt ‘Zaitsev.’
I liked his forthrightness, and tweaked him a bit by always writing him back as ‘Sasha’ no matter what the signature on the current email. By 2008, he was already well established for his work on radar astronomy in planetary science and near-Earth objects, but in the public eye he was becoming known for his broadcasts from the Evpatoria Deep Space Center in the Crimea. It was from Evpatoria that he broadcast the radio messages known as Cosmic Calls in 1999 and again in 2003. The messages were made up of audio, video, image and data files. The so-called Teen-Age Message, aimed at six Sun-like stars, went out in 2001.
Inevitably, Zaitsev became the spokesman for METI, and he defended his position with vigor in online postings as well as public debate. He had little patience with those who advised proceeding carefully, pointing out that planetary radars like Arecibo and Evpatoria were already broadcasting our presence inadvertently. To me the matter is inherently multidisciplinary, and requires the collaboration of not just physicists but historians, linguists, social scientists and more before proceeding. Zaitsev argued that planetary radars, so essential for our security against stray asteroids, were already broadcasting our presence. Should we also shut these down?
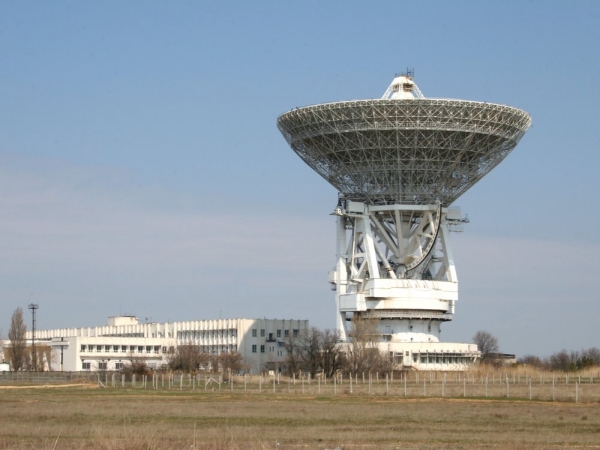
Image: RT-70 radio telescope and planetary radar at the Center for Deep Space Communications in the Crimea.
METI is a highly polarizing issue, and the arguments over intentional broadcasts continue. Surely, some argue, any advanced extraterrestrial intelligence has already picked up the signature of life on Earth, if only through analysis of our atmosphere. Some argue that our technosignature in the form of electromagnetic leakage has already entertained nearby stars with our early television shows, though Jim Benford has demonstrated that these signals are too weak to be detected by our most powerful devices. Planetary radar may indeed announce our presence — it’s strong enough to be picked up — but the counter-argument is that such beams are not aimed at specific points in space, and would be perceived as occasional transients of uncertain origin.
The debate continues, and it’s not my intention to explore it further today, so I’ll just direct those interested to several differing takes on the issue. Start with METI opponent David Brin’s article SETI, METI… and Assessing Risks like Adults, which ran in these pages in 2011, as well as Nick Nielsen’s SETI, METI and Existential Risk, from the same timeframe. Larry Klaes has an excellent overview in The Pros and Cons of METI. Remember that METI goes back to 1974 and Frank Drake’s Arecibo message, aimed at the Hercules globular cluster some 25,000 light years away and obviously symbolic.
A Declaration of Principles Concerning Activities Following the Detection of Extraterrestrial Intelligence was adopted by the International Academy of Astronautics in 1989, usually referred to simply as the First Protocol. A second protocol could have tuned up our policy for sending messages from Earth, but arguments over whether it should only affect responses to received messages — or messages sent before any extraterrestrial signal was detected — complicated the picture. The situation became so controversial that Michael Michaud and John Billingham resigned from the committee that formulated it as their language calling for international consultations was deleted.
Michaud remembered Zaitsev in an email this morning:
“I met Zaitsev at a SETI-related conference in England in 2010. He struck me as a straightforward man who spoke English well enough to engage in discussions. While I disagreed with his sending messages from a radio telescope in Ukraine without prior consultations, I had the impression that he would have been willing to talk further about the issue. I sent him a copy of my book, for which he thanked me. David Brin later initiated an email debate with Zaitsev about METI. Sasha handled David’s sharp words in a good-humored way.”
That 2010 meeting brought the two sides of the METI debate together. I want to quote Jim Benford at some length on this, as he was also involved.
“I met Alexander Zaitsev at a debate sponsored by the Royal Society in October 2010 in the UK. (The debate is documented in JBIS January 2014 volume 67 No.1 which I edited. It contains the speeches and rebuttals to the speeches). The debate was on whether sending messages to ETI should be done. Advocating METI were Seth Shostak, Stephane Dumas, and Alexander Zaitsev. The opposing team, David Brin, myself and Michael Michaud, advocated that before transmitting, a public discussion should take place to deal with the questions of who speaks for Earth and what should they say?
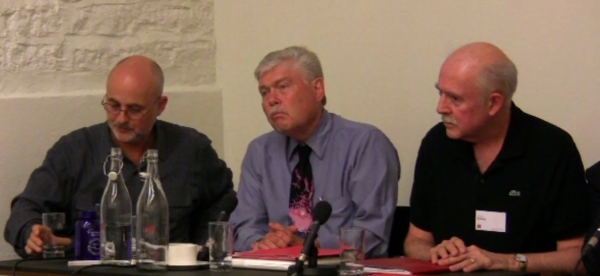
Image: (Left to right) David Brin, Jim Benford, Michael Michaud.
“Alexander Zaitsev had already transmitted messages, such as the ‘Cosmic Call 1’ message to the stars. Zaitsev radiated in 1999 from the RT-70, Evpatoria, in Crimea, Ukraine, a 70-m dish with transmitter power up to 150 kW at frequencies about 5 GHz.
“John Billingham and I pointed out that these messages were highly unlikely to be received. We took as an example the Cosmic Call 1 message. The content ranged from simple digital signals to music. Can civilizations in the stars hear them? The stars targeted ranged between 32 ly and 70 ly, so the signals will be weak when they arrive. The question then becomes: how big an antenna and how sensitive receiver electronics needed to be to detect them?
“First, we evaluated the ability of Zaitsev’s RT-70 to detect itself, assuming ETI has the same level of capability as ourselves. For a robust signal-to-noise ratio (S/N) of 10, this is 3 ly, less than the distance to even the nearest star. So the RT-70 messages would not be detected by RT-70.
“Could an ETI SKA [Square Kilometre Array] detect Earth Radio Telescopes? Zaitsev’s assumption was that Extra-Terrestrials have SKA-like systems. But for S/N=10, R=19 ly, which is not in the range of the stars targeted. Even an ET SKA would not detect the Cosmic Call 1 message.
“I presented our argument that “Who speaks for Earth?” deserves public discussion, along with our calculations.When Alexander spoke, I realized that our arguments didn’t speak to Alexander’s beliefs. He didn’t particularly care whether the messages were received. He thought it was a matter of principle to transmit. We should send messages because they announce ourselves. Reception at the other end was not necessary.”
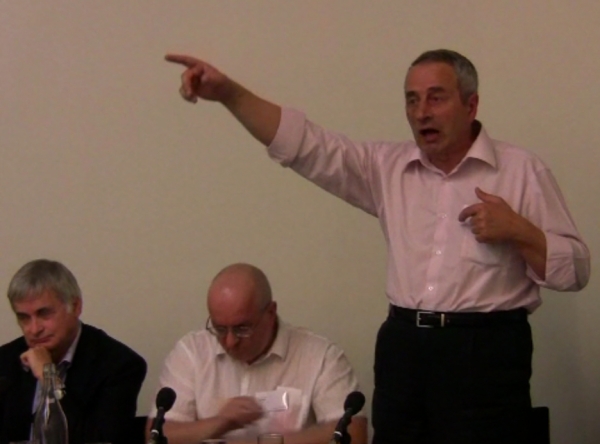
Image: A forceful Zaitsev makes a point at the Royal Society meeting. At left (left to right) are Seth Shostak and Stephane Dumas. Credit: Jim Benford.
I think Jim’s point is exactly right. In my own dealings with him, Dr. Zaitsev never made the argument that the messages he was sending would be received. I assume he looked upon them in something of the same spirit that Drake offered the Arecibo message, as a way of demonstrating the human desire to reach out into the cosmos (after all, no one would dream a message to the Hercules cluster would ever get there). But these first intentional steps to reach other civilizations would, presumably, be followed by further directed broadcasts until contact was achieved.
At least, I think that is how Dr. Zaitsev saw things. He would chafe at his inability to jump into this discussion if he were able to do so, and if I have misrepresented his view, I’m sure I would be getting one of his ‘Zaitsev’ emails rather than a friendly ‘Sasha’ signoff. But I hope I stayed on his good side most of the time. This was a man I liked and admired for his dedication despite how widely his views diverged from my own.
Asked for his thoughts on the 2010 meeting, Seth Shostak responded:
“I encountered Sasha Zaitsev at quite a few meetings, and always found him interesting and personable. He was promoting active SETI, and in that was somewhat of a lone wolf … there weren’t many who thought it was a worthy idea, and probably even fewer who thought that his transmission efforts – which he did without advance notice – were necessarily a good idea.
“But personally, I thought such criticism was kind of petty. I admired Alex for doing these things … But maybe it was because he was similar to the best scientists in boldly going …”
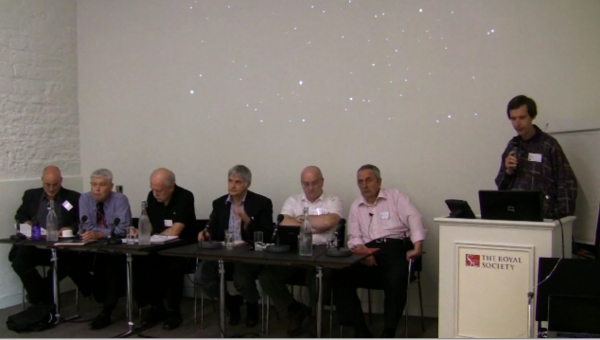
Image: The panel at the Royal Society meeting. Left to right: David Brin; Jim Benford; Michael Michaud; Seth Shostak; Stephane Dumas; Alexander Zaitsev. At podium, Martin Dominik. Credit: Jim Benford.
Alexander Zaitsev was convinced the universe held species with which we needed to engage, and I believe his purpose was to awaken the public to our potential to reach out, not in some uncertain future but right now. Given that serious METI is now joined by advertising campaigns and other private ventures, it could be said that we are not adept at presenting our best side to the cosmos, but then that too was Zaitsev’s point: It’s too late to stop this, he might have said. Let’s make our messages mean something.
David Brin, who so often engaged with him in debate, had this to say of Dr. Zaitsev:
Sasha Zaitsev was both a noted astronomer whose work in radio astronomy will long be remembered. He was also a zealous believer in a lively, beneficent cosmos. His sincere faith led him to cast forth into the heavens appeals for superior beings to offer help – or at least wisdom – to benighted (and apparently doomed) humanity. When told that it sounded a lot like ‘prayer,’ Sasha would smile. and nod. We disagreed over the wisdom or courtesy of his Yoohoo Messages, beamed from the great dish at Evpatoria, without consultation by anyone else. But if I could choose between his optimistic cosmos and the one I deem more likely, I would choose his, hands down. Perhaps – (can anyone say for sure?) – he’s finally discovered that answer.
An eloquent thought. As for me, I’ll continue to argue for informed, multidisciplinary debate and discussion in the international arena before we send further targeted messages out into the Great Silence. But in the midst of that debate, heated as it remains, I’ll miss Sasha’s voice. He probably couldn’t reach ETI even with the Evpatoria dish, but God knows he tried.
by Paul Gilster | Dec 3, 2021 | Missions |
We’ve spoken recently about civilizations expanding throughout the galaxy in a matter of hundreds of thousands of years, a thought that led Frank Tipler to doubt the existence of extraterrestrials, given the lack of evidence of such expansion. But let’s turn the issue around. What would the very beginning of our own interstellar exploration look like, if we reach the point where probes are feasible and economically viable? This is the question Johannes Lebert examines today. Johannes obtained his Master’s degree in Aerospace at the Technische Universität München (TUM) this summer. He likewise did his Bachelor’s in Mechanical Engineering at TUM and was visiting student in the field of Aerospace Engineering at the Universitat Politècnica de València (UPV), Spain. He has worked at Starburst Aerospace (a global aerospace & defense startup accelerator and strategic advisory company) and AMDC GmbH (a consultancy with focus on defense located in Munich). Today’s essay is based upon his Master thesis “Optimal Strategies for Exploring Nearby-Stars,” which was supervised by Martin Dziura (Institute of Astronautics, TUM) and Andreas Hein (Initiative for Interstellar Studies).
by Johannes Lebert
1. Introduction
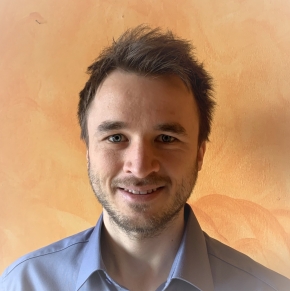
Last year, when everything was shut down and people were advised to stay at home instead of going out or traveling, I ignored those recommendations by dedicating my master thesis to the topic of interstellar travel. More precisely, I tried to derive optimal strategies for exploring near-by stars. As a very early-stage researcher I was really honored when Paul asked me to contribute to Centauri Dreams and want to thank him for this opportunity to share my thoughts on planning interstellar exploration from a strategic perspective.
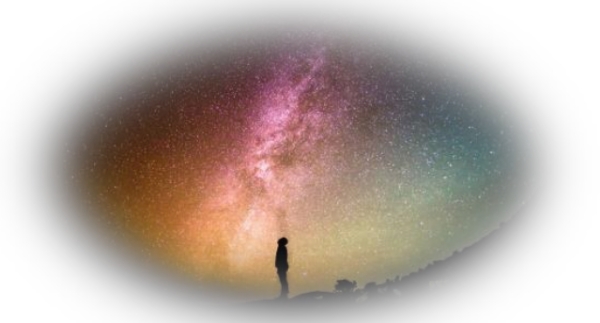
Figure 1: Me, last year (symbolic image). Credit: hippopx.com).
As you are an experienced and interested reader of Centauri Dreams, I think it is not necessary to make you aware of the challenges and fascination of interstellar travel and exploration. I am sure you’ve already heard a lot about interstellar probe concepts, from gram-scale nanoprobes such as Breakthrough Starshot to huge spaceships like Project Icarus. Probably you are also familiar with suitable propulsion technologies, be it solar sails or fusion-based engines. I guess, you could also name at least a handful of promising exploration targets off the cuff, perhaps with focus on star systems that are known to host exoplanets. But have you ever thought of ways to bring everything together by finding optimal strategies for interstellar exploration? As a concrete example, what could be the advantages of deploying a fleet of small probes vs. launching only few probes with respect to the exploration targets? And, more fundamentally, what method can be used to find answers to this question?
In particular the last question has been the main driver for this article: Before starting with writing, I was wondering a lot what could be the most exciting result I could present to you and found that the methodology as such is the most valuable contribution on the way towards interstellar exploration: Once the idea is understood, you are equipped with all relevant tools to generate your own results and answer similar questions. That is why I decided to present you a summary of my work here, addressing more directly the original idea of Centauri Dreams (“Planning […] Interstellar Exploration”), instead of picking a single result.
Below you’ll find an overview of this article’s structure to give you an impression of what to expect. Of course, there is no time to go into detail for each step, but I hope it’s enough to make you familiar with the basic components and concepts.
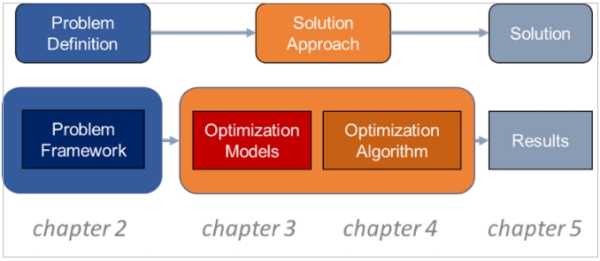
Figure 2: Article content and chapters
I’ll start from scratch by defining interstellar exploration as an optimization problem (chapter 2). Then, we’ll set up a model of the solar neighborhood and specify probe and mission parameters (chapter 3), before selecting a suitable optimization algorithm (chapter 4). Finally, we apply the algorithm to our problem and analyze the results (more generally in chapter 5, with implications for planning interstellar exploration in chapter 6).
But let’s start from the real beginning.
2. Defining and Classifying the Problem of Interstellar Exploration
We’ll start by stating our goal: We want to explore stars. Actually, it is star systems, because typically we are more interested in the planets that are potentially hosted by a star instead of the star as such. From a more abstract perspective, we can look at the stars (or star systems) as a set of destinations that can be visited and explored. As we said before, in most cases we are interested in planets orbiting the target star, even more if they might be habitable. Hence, there are star systems which are more interesting to visit (e. g. those with a high probability of hosting habitable planets) and others, which are less attracting. Based on these considerations, we can assign each star system an “earnable profit” or “stellar score” from 0 to 1. The value 0 refers to the most boring star systems (though I am not sure if there are any boring star systems out there, so maybe it’s better to say “least fascinating”) and 1 to the most fascinating ones. The scoring can be adjusted depending on one’s preferences, of course, and extended by additional considerations and requirements. However, to keep it simple, let’s assume for now that each star system provides a score of 1, hence we don’t distinguish between different star systems. Having this in mind, we can draw a sketch of our problem as shown in Figure 3.
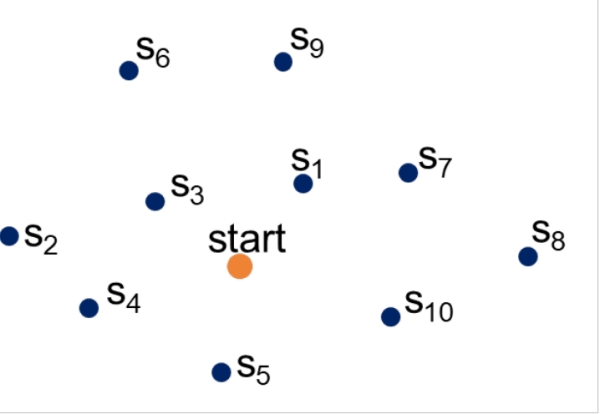
Figure 3: Solar system (orange dot) as starting point, possible star systems for exploration (destinations with score ) represented by blue dots
To earn the profit by visiting and exploring those destinations, we can deploy a fleet of space probes, which are launched simultaneously from Earth. However, as there are many stars to be explored and we can only launch a limited number of probes, one needs to decide which stars to include and which ones to skip – otherwise, mission timeframes will explode. This decision will be based on two criteria: Mission return and mission duration. The mission return is simply the sum of the stellar score of each visited star. As we assume a stellar score of 1 for each star, the mission return is equal to the number of stars that is visited by all our probes. The mission duration is the time needed to finish the exploration mission.
In case we deploy several probes, which carry out the exploration mission simultaneously, the mission is assumed to be finished when the last probe reaches the last star on its route – even if other probes have finished their route earlier. Hence, the mission duration is equal to the travel time of the probe with the longest trip. Note that the probes do not need to return to the solar system after finishing their route, as they are assumed to send the data gained during exploration immediately back to Earth.
Based on these considerations we can classify our problem as a bi-objective multi-vehicle open routing problem with profits. Admittedly quite a cumbersome term, but it contains all relevant information:
- Bi-objective: There are two objectives, mission return and mission duration. Note that we want to maximize the return while keeping the duration minimal. Hence, from intuition we can expect that both objectives are competing: The more time, the more stars can be visited.
- Multi-vehicle: Not only one, but several probes are used for simultaneous exploration.
- Open: Probes are free to choose where to end their route and are not forced to return back to Earth after finishing their exploration mission.
- Routing problem with profits: We consider the stars as a set of destinations with each providing a certain score si. From this set, we need to select several subsets, which are arranged as routes and assigned to different probes (see Figure 4).
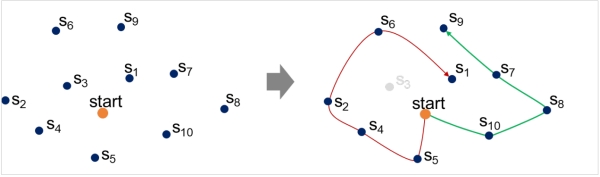
Figure 4: Problem illustration: Identify subsets of possible destinations si, find the best sequences and assign them to probes
Even though it appears a bit stiff, the classification of our problem is very useful to identify suitable solution methods: Before, we were talking about the problem of optimizing interstellar exploration, which is quite unknown territory with limited research. Now, thanks to our abstraction, we are facing a so-called Routing Problem, which is a well-known optimization problem class, with several applications across various fields and therefore being exhaustively investigated. As a result, we now have access to a large pool of established algorithms, which have already been tested successfully against these kinds of problems or other very similar or related problems such as the Traveling Salesman Problem (probably the most popular one) or the Team Orienteering Problem (subclass of the Routing Problem).
3. Model of the Solar Neighborhood and Assumptions on Probe & Mission Architecture
Obviously, we’ll also need some kind of galactic model of our region of interest, which provides us with the relevant star characteristics and, most importantly, the star positions. There are plenty of star catalogues with different focus and historical background (e.g. Hipparcos, Tycho, RECONS). One of the latest, still ongoing surveys is the Gaia Mission, whose observations are incorporated in the Gaia Archive, which is currently considered to be the most complete and accurate star database.
However, the Gaia Archive – more precisely the Gaia Data Release 2 (DR2), which will be used here* (accessible online [1] together with Gaia based distance estimations by Bailer-Jones et al. [2]) – provides only raw observation data, which include some reported spurious results. For instance, it lists more than 50 stars closer than Proxima Centauri, which would be quite a surprise to all the astronomers out there.
*1. Note that there is already an updated Data Release (Gaia DR3), which was not available yet at the time of the thesis.
Hence, a filtering is required to obtain a clean data set. The filtering procedure applied here, which consists of several steps, is illustrated in Figure 5 and follows the suggestions from Lindegren et al. [3]. For instance, data entries are eliminated based on parallax errors and uncertainties in BP and RP fluxes. The resulting model (after filtering) includes 10,000 stars and represents a spherical domain with a radius of roughly 110 light years around the solar system.
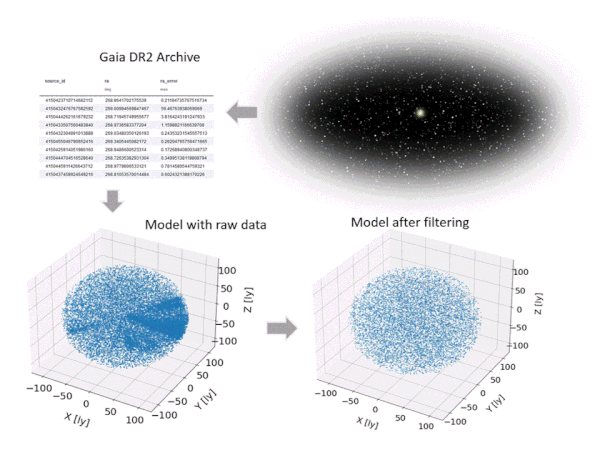
Figure 5: Setting up the star model based on Gaia DR2 and filtering (animated figure from [9])
To reduce the complexity of the model, we assume all stars to maintain fixed positions – which is of course not true (see Figure 5 upper right) but can be shown to be a valid simplification for our purposes, and we limit the mission time frames to 7,000 years. 7,000 years? Yes, unfortunately, the enormous stellar distances, which are probably the biggest challenge we encounter when planning interstellar travel, result in very high travel times – even if we are optimistic concerning the travel speed of our probes, which are defined by the following.
We’ll use a rather simplistic probe model based on literature suggestions, which has the advantage that the results are valid across a large range of probe concepts. We assume the probes to travel along straight-line trajectories (in line with Fantino & Casotto [4] at an average velocity of 10 % of the speed of light (in line with Bjørk [5]. They are not capable of self-replicating; hence, the probe number remains constant during a mission. Furthermore, the probes are restricted to performing flybys instead of rendezvous, which limits the scientific return of the mission but is still good enough to detect planets (as reported by Crawford [6]. Hence, the considered mission can be interpreted as a reconnaissance or scouting mission, which serves to identify suitable targets for a follow-up mission, which then will include rendezvous and deorbiting for further, more sophisticated exploration.
Disclaimer: I am well aware of the weaknesses of the probe and mission model, which does not allow for more advanced mission design (e. g. slingshot maneuvers) and assumes a very long-term operability of the probes, just to name two of them. However, to keep the model and results comprehensive, I tried to derive the minimum set of parameters which is required to describe interstellar exploration as an optimization problem. Any extensions of the model, such as a probe failure probability or deorbiting maneuvers (which could increase the scientific return tremendously), are left to further research.
4. Optimization Method
Having modeled the solar neighborhood and defined an admittedly rather simplistic probe and mission model, we finally need to select a suitable algorithm for solving our problem, or, in other words, to suggest “good” exploration missions (good means optimal with respect to both our objectives). In fact, the algorithm has the sole task of assigning each probe the best star sequences (so-called decision variables). But which algorithm could be a good choice?
Optimization or, more generally, operations research is a huge research field which has spawned countless more or less sophisticated solution approaches and algorithms over the years. However, there is no optimization method (not yet) which works perfectly for all problems (“no free lunch theorem”) – which is probably the main reason why there are so many different algorithms out there. To navigate through this jungle, it helps to recall our problem class and focus on the algorithms which are used to solve equal or similar problems. Starting from there, we can further exclude some methods a priori by means of a first analysis of our problem structure: Considering n stars, there are ?! possibilities to arrange them into one route, which can be quite a lot (just to give you a number: for n=50 we obtain 50!? 1064 possibilities).
Given that our model contains up to 10,000 stars, we cannot simply try out each possibility and take the best one (so called enumeration method). Instead, we need to find another approach, which is more suitable for those kinds of problems with a very large search space, as an operations researcher would say. Maybe you already have heard about (meta-)heuristics, which allow for more time-efficient solving but do not guarantee to find the true optimum. Even if you’ve never heard about them, I am sure that you know at least one representative of a metaheuristic-based solution, as it is sitting in front of your screen right now as you are reading this article… Indeed, each of us is the result of a thousands of years lasting, still ongoing optimization procedure called evolution. Wouldn’t it be cool if we could adopt the mechanisms that brought us here to do the next, big step in mankind and find ways to leave the solar system and explore unknown star systems?
Those kinds of algorithms, which try to imitate the process of natural evolution, are referred to as Genetic Algorithms. Maybe you remember the biology classes at school, where you learned about chromosomes, genes and how they are shared between parents and their children. We’ll use the same concept and also the wording here, which is why we need to encode our optimization problem (illustrated in Figure 6): One single chromosome will represent one exploration mission and as such one possible solution for our optimization problem. The genes of the chromosome are equivalent to the probes. And the gene sequences embody the star sequences, which in turn define the travel routes of each probe.
If we are talking about a set of chromosomes, we will use the term “population”, therefore sometimes one chromosome is referred to as individual. Furthermore, as the population will evolve over the time, we will speak about different generations (just like for us humans).
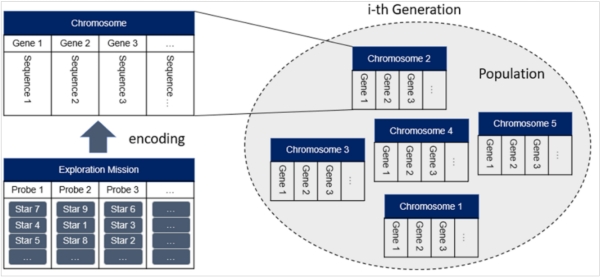
Figure 6. Genetic encoding of the problem: Chromosomes embody exploration missions; genes represent probes and gene sequences are equivalent to star sequences.
The algorithm as such is pretty much straightforward, the basic working principle of the Genetic Algorithm is illustrated below (Figure 7). Starting from a randomly created initial population, we enter an evolution loop, which stops either when a maximum number of generations is reached (one loop represents one generation) or if the population stops evolving and keeps stable (convergence is reached).
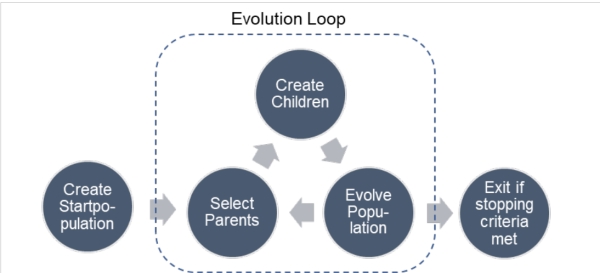
Figure 7: High level working procedure of the Genetic Algorithm
I don’t want to go into too much detail on the procedure – interested readers are encouraged to go through my thesis [7] and look for the corresponding chapter or see relevant papers (particularly Bederina and Hifi [8], from where I took most of the algorithm concept). To summarize the idea: Just like in real life, chromosomes are grouped into pairs (parents) and create children (representing new exploration missions) by sharing their best genes (which are routes in our case). For higher variety, a mutation procedure is applied to a few children, such as a partial swap of different route segments. Finally, the worst chromosomes are eliminated (evolve population = “survival of the fittest”) to keep the population size constant.
Side note: Currently, we have the chance to observe this optimization procedure when looking at the Coronavirus. It started almost two years ago with the alpha version; right now the population is dominated by the delta version, with omicron an emerging variant. From the virus perspective, it has improved over time through replication and mutation, which is supported by large populations (i.e., a high number of cases).
Note that the genetic algorithm is extended by a so-called local search, which comprises a set of methods to improve routes locally (e. g. by inverting segments or swapping two random stars within one route). That is why this method is referred to as Hybrid Genetic Algorithm.
Now let’s see how the algorithm is operating when applied to our problem. In the animated figure below, we can observe the ongoing optimization procedure. Each individual is evaluated “live” with respect to our objectives (mission return and duration). The result is plotted in a chart, where one dot refers to one individual and thus represents one possible exploration mission. The color indicates the corresponding generation.
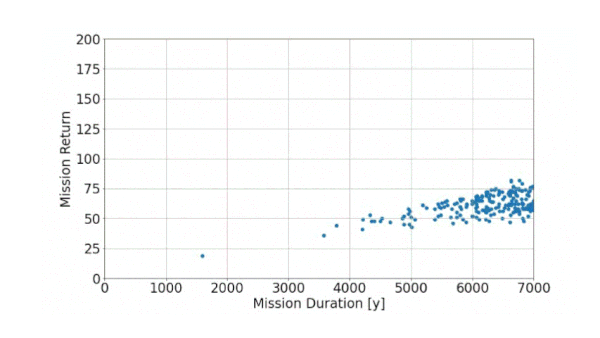
Figure 8: Animation of the ongoing optimization procedure: Each individual (represented by a dot) is evaluated with respect to the objectives, one color indicates one generation
As shown in this animated figure, the algorithm seems to work properly: With increasing generations, it tries to generate better solutions, as it optimizes towards higher mission return and lower mission duration (towards the upper left in the Figure 8). Solutions from the earlier generation with poor quality are subsequently replaced by better individuals.
5. Optimization Results
As a result of the optimization, we obtain a set of solutions (representing the surviving individuals from the final generation), which build a curve when evaluated with respect to our twin objectives of mission duration and return (see Figure 9). Obviously, we’ll get different curves when we change the probe number m between two optimization runs. In total, 9 optimization runs are performed; after each run the probe number is doubled, starting with m=2. As already in the animated Figure 8, one dot represents one chromosome and thus one possible exploration mission (one mission is illustrated as an example).
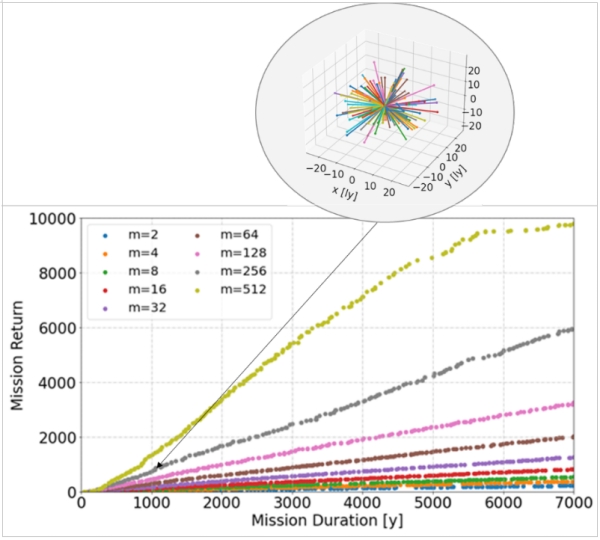
Figure 9: Resulting solutions for different probe numbers and mission example represented by one dot
Already from this plot, we can make some first observations: The mission return (which we assume equal to the number of explored stars, just as a reminder) increases with mission duration. More precisely, there appears to be an approximately linear incline of star number with time, at least in most instances. This means that when doubling the mission duration, we can expect more or less twice the mission return. An exception to this behavior is the 512 probes curve, which flattens when reaching > 8,000 stars due to the model limits: In this region, only few unexplored stars are left which may require unfavorable transfers.
Furthermore, we see that for a given mission duration the number of explored stars can be increased by launching more probes, which is not surprising. We will elaborate a bit more on the impact of the probe number and on how it is linked with the mission return in a minute.
For now, let’s keep this in our mind and take a closer look at the missions suggested by the algorithm. In the figure below (Figure 10), routes for two missions with different probe number m but similar mission return J1 (nearly 300 explored stars) are visualized (x, y, z-axes dimensions in light years). One color indicates one route that is assigned to one probe.
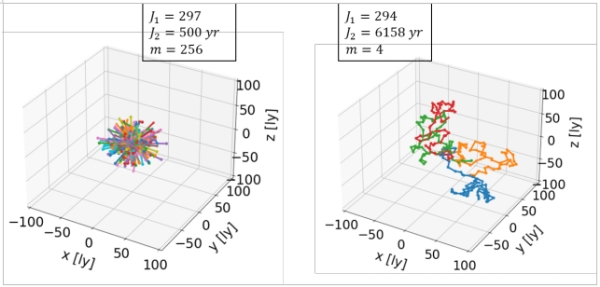
Figure 10: Visualization of two selected exploration missions with similar mission return J1 but different probe number m – left: 256 available probes, right: 4 available probes (J2 is the mission duration in years)
Even though the mission return is similar, the route structures are very different: The higher probe number mission (left in Figure 10) is built mainly from very dense single-target routes and thus focuses more on the immediate solar neighborhood. The mission with only 4 probes (right in Figure 10), contrarily, contains more distant stars, as it consists of comparatively long, chain-like routes with several targets included. This is quite intuitive: While for the right case (few probes available) mission return is added by “hopping” from star to star, in the left case (many probes available) simply another probe is launched from Earth. Needless to say, the overall mission duration J2 is significantly higher when we launch only 4 probes (> 6000 years compared to 500 years).
Now let’s look a bit closer at the corresponding transfers. As before, we’ll pick two solutions with different probe number (4 and 64 probes) and similar mission return (about 230 explored stars). But now, we’ll analyze the individual transfer distances along the routes instead of simply visualizing the routes. This is done by means of a histogram (shown in Figure 11), where simply the number of transfers with a certain distance is counted.
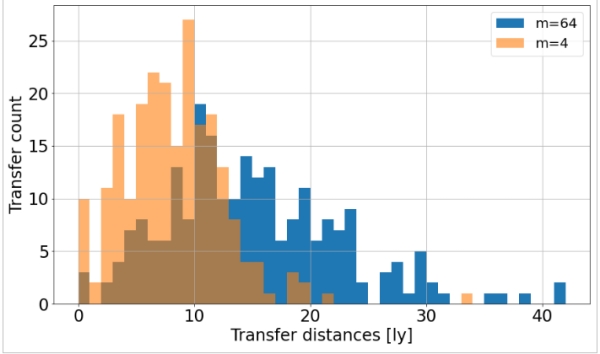
Figure 11: Histogram with transfer distances for two different solution – orange bars belong to a solution with 4 probes, blue bars to a solution with 64 probes; both provide a mission return of roughly 230 explored stars.
The orange bars belong to a solution with 4 probes, the blue ones to a solution with 64 probes. To give an example on how to read the histogram: We can say that the solution with 4 probes includes 27 transfers with a distance of 9 light years, while the solution with 64 probes contains only 8 transfers of this distance. What we should take from this figure is that with higher probe numbers apparently more distant transfers are required to provide the same mission return.
Based on this result we can now concretize earlier observations regarding the probe number impact: From Figure 9 we already found that the mission return increases with probe number, without being more specific. Now, we discovered that the efficiency of the exploration mission w. r. t. routing decreases with increasing probe number, as there are more distant transfers required. We can even quantify this effect: After doing some further analysis on the result curve and a bit of math, we’ll find that the mission return J1 scales with probe number m according to ~m0.6 (at least in most instances). By incorporating the observations on linearity between mission return and duration (J2), we obtain the following relation: J1 ~ J2m0.6.
As J1 grows only with m0.6 (remember that m1 indicates linear growth), the mission return for a given mission duration does not simply double when we launch twice as many probes. Instead, it’s less; moreover, it depends on the current probe number – in fact, the contribution of additional probes to the overall mission return diminishes with increasing probe numbers.
This phenomenon is similar to the concept of diminishing returns in economics, which denotes the effect that an increase of the input yields progressively lower or even reduced increase in output. How does that fit with earlier observations, e. g. on route structure? Apparently, we are running into some kind of a crowding effect, when we launch many probes from the same spot (namely our solar system): Long initial transfers are required to assign each probe an unexplored star. Obviously, this effect intensifies with each additional probe being launched.
6. Conclusions and Implications for Planning Interstellar Exploration
What can we take from all this effort and the results of the optimization? First, let’s recap the methodology and tools which we developed for planning interstellar exploration (see Figure 12).
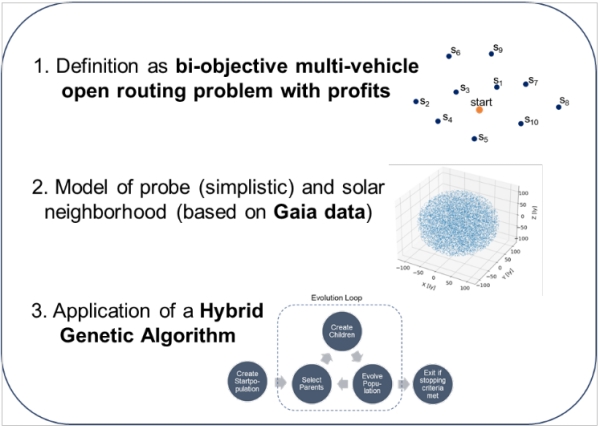
Figure 12: Methodology – main steps
Beside the methodology, which of course can be extended and adapted, we can give some recommendations for interstellar mission design considerations, in particular regarding the probe number impact:
- High probe numbers are favorable when we want to explore many stars in the immediate solar neighborhood. As further advantage of high probe numbers, mostly single-target missions are performed, which allows the customization of each probe according to its target star (e. g. regarding scientific instrumentation).
- If the number of available probes is limited (e. g. due to high production costs), it is recommended to include more distant stars, as it enables a more efficient routing. The aspect of higher routing efficiency needs to be considered in particular when fuel costs are relevant (i. e. when fuel needs to be transported aboard). For other, remotely propelled concepts (such as laser driven probes, e. g. Breakthrough Starshot) this issue is less relevant, which is why those concepts could be deployed in larger numbers, allowing for shorter overall mission duration at the expense of more distant transfers.
- When planning to launch a high number of probes from Earth, however, one should be aware of crowding effects. This effect sets in already for few probes and intensifies with each additional probe. One option to encounter this issue and thus support a more efficient probe deployment could be swarm-based concepts, as indicated by the sketch in Figure 13.
The swarm-based concept includes a mother ship, which transports a fleet of smaller explorer probes to a more distant star. After arrival, the probes are released and start their actual exploration mission. As a result, the very dense, crowded route structures, which are obtained when many probes are launched from the same spot (see again Figure 10, left plot), are broken up.
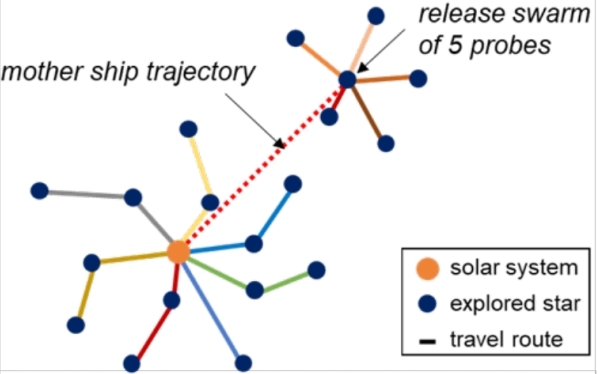
Figure 13: Sketch illustrating the beneficial effect of swarm concepts for high probe numbers.
Obviously, the results and derived implications for interstellar exploration are not mind-blowing, as they are mostly in line with what one would expect. However, this in turn indicates that our methodology seems to work properly, which of course does not serve as a full verification but is at least a small hint. A more reliable verification result can be obtained by setting up a test problem with known optimum (which is not shown here, but was also done for this approach, showing that the algorithm’s results deviate about 10% compared to the ideal solution).
Given the very early-stage level of this work, there is still a lot of potential for further research and refinement of the simplistic models. Just to pick one example: As a next step, one could start to distinguish between different star systems by varying the reward of each star system si based on a stellar metric, where more information of the star is incorporated (such as spectral class, metallicity, data quality, …). In the end it’s up to oneself, which questions he or she wants to answer – there is more than enough inspiration up there in the night sky.
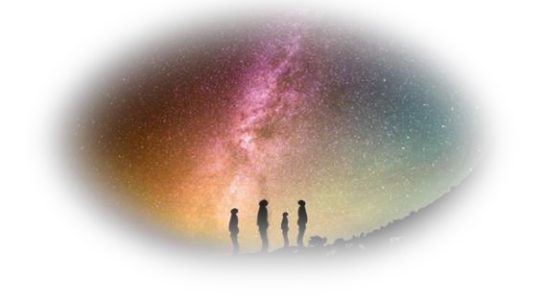
Figure 14: More people, now
Assuming that you are not only an interested reader of Centauri Dreams but also familiar with other popular literature on that topic, you maybe have heard about Clarke’s three laws. I would like to close this article by taking up his second one: The only way of discovering the limits of the possible is to venture a little way past them into the impossible. As said before, I hope that the introduced methodology can help to answer further questions concerning interstellar exploration from a strategic perspective. The more we know, the better we are capable of planning and imagining interstellar exploration, thus pushing gradually the limits of what is considered to be possible today.
References
[1] ESA, “Gaia Archive,“ [Online]. Available: https://gea.esac.esa.int/archive/.
[2] C. A. L. Bailer-Jones et al., “Estimating Distances from Parallaxes IV: Distances to 1.33 Billion Stars in Gaia Data Release 2,” The Astronomical Journal, vol. 156, 2018.
https://iopscience.iop.org/article/10.3847/1538-3881/aacb21
[3] L. Lindegren et al., “Gaia Data Release 2 – The astrometric solution,” Astronomy & Astrophysics, vol. 616, 2018.
https://doi.org/10.1051/0004-6361/201832727
[4] E. Fantino and S. Casotto, “Study on Libration Points of the Sun and the Interstellar Medium for Interstellar Travel,” Universitá di Padova/ESA, 2004.
[5] R. Bjørk, “Exploring the Galaxy using space probes,” International Journal of Astrobiology, vol. 6, 2007.
https://doi.org/10.1017/S1473550407003709
[6] I. A. Crawford, “The Astronomical, Astrobiological and Planetary Science Case for Interstellar Spaceflight,” Journal of the British Interplanetary Society, vol. 62, 2009. https://arxiv.org/abs/1008.4893
[7] J. Lebert, “Optimal Strategies for Exploring Near-by Stars,“ Technische Universität München, 2021.
https://mediatum.ub.tum.de/1613180
[8] H. Bederina and M. Hifi, “A Hybrid Multi-Objective Evolutionary Algorithm for the Team Orienteering Problem,” 4th International Conference on Control, Decision and Information Technologies, Barcelona, 2017.
https://ieeexplore.ieee.org/document/8102710
[9] University of California – Berkeley, “New Map of Solar Neighborhood Reveals That Binary Stars Are All Around Us,” SciTech Daily, 22 February 2021.
https://scitechdaily.com/new-map-of-solar-neighborhood-reveals-that-binary-stars-are-all-around-us/
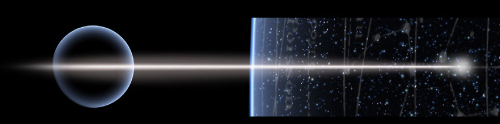